In the rapidly evolving world of artificial intelligence, DLNet has emerged as a groundbreaking solution that is reshaping the way we approach deep learning. As technology continues to advance, DLNet stands out as a crucial framework that enhances efficiency, accuracy, and scalability in AI models. This article delves into the intricacies of DLNet, its applications, and its potential to revolutionize industries.
DLNet is not just another framework; it represents a significant leap forward in deep learning technology. By addressing the limitations of traditional models, DLNet offers a more robust and versatile solution for developers and researchers. Its innovative architecture allows for faster processing, greater accuracy, and improved adaptability across various domains.
As we explore the capabilities of DLNet, it becomes evident that its impact extends beyond the tech industry. From healthcare to finance, DLNet's applications promise to transform how businesses and organizations operate, making processes more efficient and intelligent. This article aims to provide a comprehensive understanding of DLNet, ensuring that readers are equipped with the knowledge to harness its potential.
Read also:Hdhub4u In Movies Bollywood Your Ultimate Guide To Highquality Bollywood Entertainment
What is DLNet?
DLNet, or Deep Learning Network, is an advanced framework designed to simplify and enhance the development of deep learning models. It serves as a powerful tool for researchers and developers, enabling them to create sophisticated AI applications with ease. DLNet's architecture is built on the principles of scalability, flexibility, and performance, making it an ideal choice for complex machine learning tasks.
One of the key features of DLNet is its ability to integrate seamlessly with existing technologies. This compatibility ensures that businesses can adopt DLNet without overhauling their current systems. Additionally, DLNet supports multiple programming languages, including Python and C++, allowing developers to choose the language that best suits their needs.
Key Features of DLNet
- Scalability: DLNet can handle large datasets and complex models, making it suitable for enterprise-level applications.
- Flexibility: The framework supports a wide range of neural network architectures, enabling developers to experiment with different models.
- Performance: DLNet's optimized algorithms ensure faster training times and improved accuracy in predictions.
- Compatibility: It integrates with popular libraries and tools, enhancing its usability across various platforms.
Applications of DLNet in Various Industries
The versatility of DLNet makes it applicable in numerous industries, each benefiting from its unique capabilities. From healthcare to finance, DLNet's applications are transforming traditional processes and driving innovation. Below, we explore some of the key industries where DLNet is making a significant impact.
Healthcare
In the healthcare sector, DLNet is revolutionizing diagnostics and treatment planning. By analyzing vast amounts of medical data, DLNet helps in identifying patterns and predicting outcomes with remarkable accuracy. This capability is crucial in early detection of diseases and personalized treatment strategies.
Finance
DLNet's application in finance is reshaping risk assessment and fraud detection. Its ability to process large volumes of financial data enables institutions to identify potential risks and anomalies in real-time. This proactive approach enhances security and ensures compliance with regulatory standards.
Retail
For retailers, DLNet enhances customer experience through personalized recommendations and inventory management. By analyzing consumer behavior and market trends, DLNet helps businesses make informed decisions, leading to increased sales and customer satisfaction.
Read also:Perdita Weeks Disability A Comprehensive Guide To Understanding Her Journey
Advantages of Using DLNet
DLNet offers several advantages that set it apart from other deep learning frameworks. These benefits contribute to its growing popularity among developers and researchers worldwide. Below are some of the key advantages of DLNet:
- Enhanced Efficiency: DLNet's optimized algorithms reduce training times, allowing for faster development cycles.
- Improved Accuracy: The framework's advanced architecture ensures higher accuracy in predictions, leading to better decision-making.
- Cost-Effective: By reducing the need for expensive hardware, DLNet lowers the overall cost of developing and deploying AI models.
- Community Support: DLNet benefits from a vibrant community of developers and researchers, providing continuous updates and improvements.
Challenges and Limitations of DLNet
Despite its numerous advantages, DLNet is not without its challenges. Understanding these limitations is crucial for maximizing its potential and overcoming obstacles. Below are some of the key challenges associated with DLNet:
- Complexity: DLNet's advanced architecture can be difficult for beginners to grasp, requiring extensive training and expertise.
- Resource Intensive: While DLNet optimizes performance, it still requires significant computational resources for large-scale applications.
- Learning Curve: Developers transitioning from other frameworks may face a steep learning curve when adopting DLNet.
DLNet vs. Other Deep Learning Frameworks
When compared to other deep learning frameworks, DLNet stands out due to its unique features and capabilities. Below is a comparison of DLNet with popular frameworks such as TensorFlow and PyTorch:
TensorFlow
TensorFlow is widely regarded as one of the most comprehensive deep learning frameworks. However, DLNet offers greater flexibility and scalability, making it a better choice for complex applications. Additionally, DLNet's optimized algorithms ensure faster training times, providing a competitive edge.
PyTorch
PyTorch is known for its ease of use and dynamic computation graph. While DLNet shares some of these features, it excels in performance and efficiency, particularly for large-scale applications. DLNet's compatibility with multiple programming languages also gives it an advantage over PyTorch.
Future Developments and Innovations in DLNet
The future of DLNet is promising, with ongoing research and development aimed at enhancing its capabilities. Key areas of focus include improving scalability, reducing computational requirements, and expanding its range of applications. Below are some of the anticipated innovations in DLNet:
- Quantum Computing Integration: DLNet is exploring the potential of quantum computing to enhance its performance and efficiency.
- Edge Computing Support: Future versions of DLNet will include support for edge computing, enabling real-time processing on devices.
- AutoML Integration: DLNet is incorporating AutoML capabilities to automate the model-building process, making it more accessible to non-experts.
Implementing DLNet in Your Projects
Implementing DLNet in your projects involves several steps, from setting up the environment to deploying the model. Below is a step-by-step guide to help you get started:
Setting Up the Environment
Before you begin, ensure that your system meets the necessary requirements. Install the latest version of DLNet and configure your development environment to support its features. This setup process is straightforward and well-documented in the official DLNet documentation.
Building and Training Models
Once the environment is ready, you can start building and training your models. DLNet provides a wide range of pre-built models and datasets to help you get started quickly. Additionally, its intuitive interface simplifies the process of creating custom models tailored to your specific needs.
Expert Insights and Case Studies
To gain a deeper understanding of DLNet's capabilities, it is helpful to explore expert insights and real-world case studies. Below are some examples of how DLNet is being used in various industries:
Case Study: Healthcare
A leading healthcare provider implemented DLNet to enhance its diagnostic capabilities. By analyzing patient data, DLNet helped in identifying potential health risks and recommending personalized treatment plans. This application resulted in improved patient outcomes and reduced costs.
Case Study: Finance
A major financial institution adopted DLNet to strengthen its fraud detection system. The framework's ability to process large volumes of data in real-time enabled the institution to identify suspicious activities promptly, enhancing security and compliance.
Conclusion
In conclusion, DLNet represents a significant advancement in deep learning technology, offering unparalleled capabilities for developers and researchers. Its applications across various industries highlight its versatility and potential to drive innovation. By understanding its features, advantages, and limitations, businesses can harness the power of DLNet to enhance their operations and achieve greater success.
We invite you to share your thoughts and experiences with DLNet in the comments section below. Additionally, explore our other articles to learn more about cutting-edge technologies and their applications. Together, let's shape the future of artificial intelligence!
Table of Contents
- What is DLNet?
- Key Features of DLNet
- Applications of DLNet in Various Industries
- Healthcare
- Finance
- Retail
- Advantages of Using DLNet
- Challenges and Limitations of DLNet
- DLNet vs. Other Deep Learning Frameworks
- Future Developments and Innovations in DLNet
- Implementing DLNet in Your Projects
- Expert Insights and Case Studies
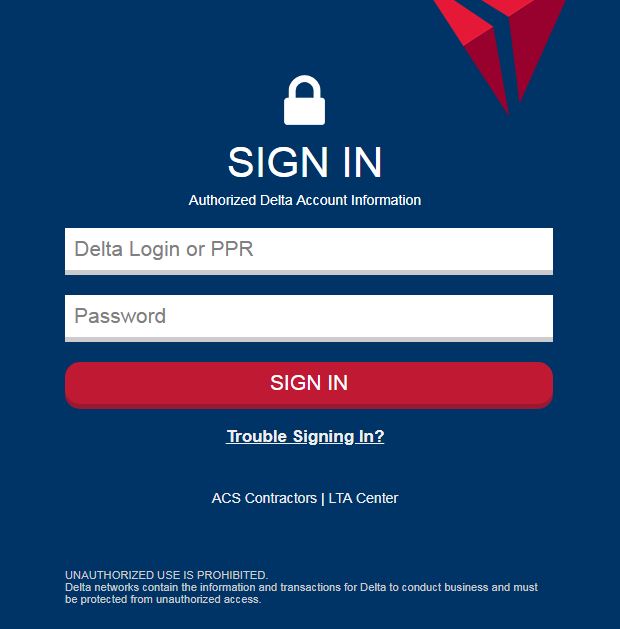
